Machine learning study looks at younger population to identify, mitigate cardiometabolic risks
Binghamton researcher to lead $2.5 million project for the National Institutes of Health
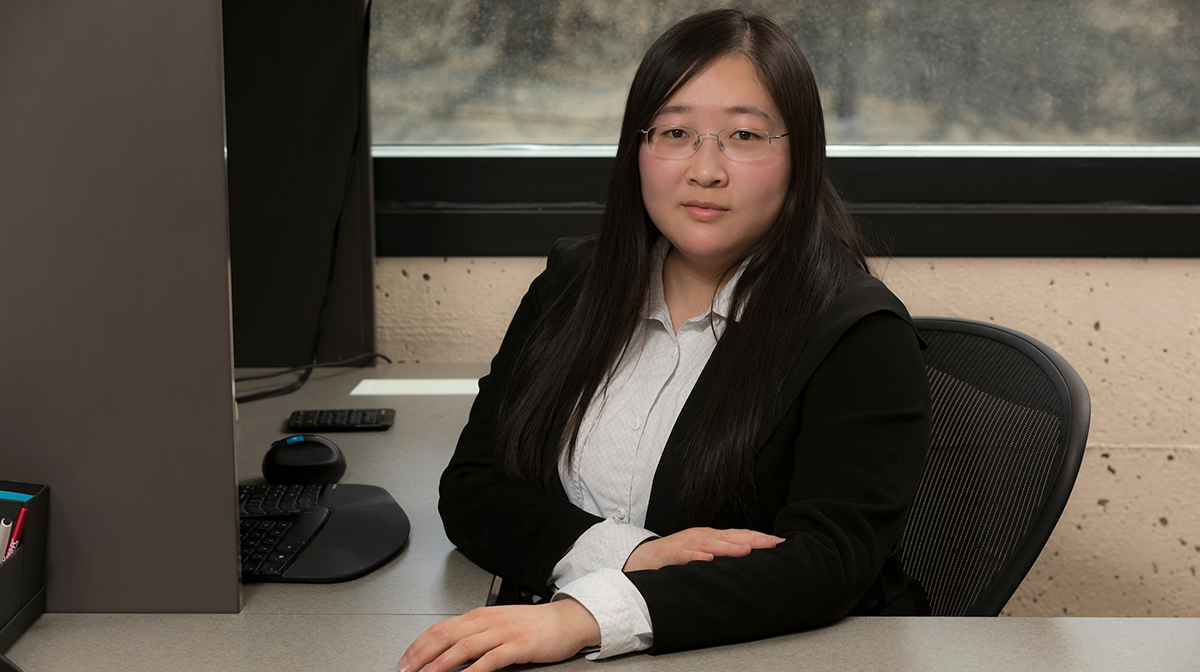
Cardiometabolic diseases are the top cause of preventable deaths worldwide, and the number of people who experience one or more of these conditions during their lifetime is increasing.
Still, much of the research about these diseases has focused on the adult and senior populations. What if younger people and the healthcare professionals who treat them could better understand the risk factors that lead to health problems later in life and reduce these factors in advance?
That’s the thinking behind new research led by Assistant Professor Bing Si from Binghamton University’s Thomas J. Watson College of Engineering and Applied Science. Working in collaboration with clinical scientists from Mayo Clinic and Harvard University, Si will develop novel statistical machine models to analyze thousands of young individuals’ health data — anonymized, of course — and predict cardiometabolic risks in adolescents and young adults.
Among the risk factors to be tracked will be metabolic dysregulation, obesity, physical inactivity, poor nutrition, sleep disorders and other related conditions that can lead to a higher chance of severe cardiometabolic outcomes, such as cardiovascular morbidity and mortality. Existing data show that many of these risk factors disproportionally affect the underrepresented minority population, resulting in health disparities.
The five-year project recently received a $2.5 million R01 award from the National Institutes of Health, with $1.8 million coming directly to Binghamton.
“My research is on statistical modeling and machine learning with a focus on multimodal health data analysis, and these data can have very complex structures and challenging properties,” said Si, a faculty member in the Department of Systems Science and Industrial Engineering. “I am working to develop new data fusion and machine learning models that tackle these challenges in data analysis and generate new knowledge to facilitate medical decision-making. In this project, we have this large data set with thousands of individuals to identify those high-risk versus low-risk subgroups from the young population.”
Among the data being analyzed are socio-demographics, dietary information, blood tests, sleep studies, exercise habits, health questionnaires, medical checkups and other information.
“One big challenge is that there is missingness,” Si said. “If you are collecting multimodal data from thousands of people, for sure somebody will miss something. Some tests may be unreliable and we cannot use them. We are trying to use a statistical modeling approach to address that as well.”
While Si’s group is leading the model development and application, her collaborators from Harvard and Mayo Clinic are contributing valuable knowledge and medical insight to aid this research. “This project would not be possible without the teamwork between industrial system engineers and medical professionals,” she said.
By the end of the five-year grant, Si hopes that her study will generate insight into different cardiometabolic subgroups that can help not only with treatment but also early intervention for high-risk groups. Her methodological framework could also be used to study other complex diseases to facilitate precision medicine and promote population health.
“This is not the job of one grant to do, but we hope that after we complete our R01 project, we can contribute some new knowledge to the field and continue to study this area,” she said. “Our overarching goal is to improve cardiometabolic healthcare in young people as they transition into adulthood, and eventually to reduce the health disparity in diverse populations and reduce healthcare costs in the U.S.”